In this session Mike Ferguson looks at different architectures that recent were offered by many different vendors claiming to be ‘the modern data architecture solution’ for the data-driven enterprise, with support for open table formats such as Apache Iceberg, Apache Hudi and Delta Lake. In addition, we have seen significant new milestones in extending the ISO SQL Standard to support new kinds of analytics in general purpose SQL. He will discuss the impact of this on analytical data platforms and what it means for customers.
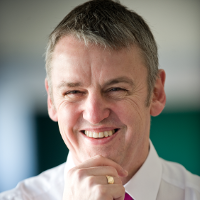